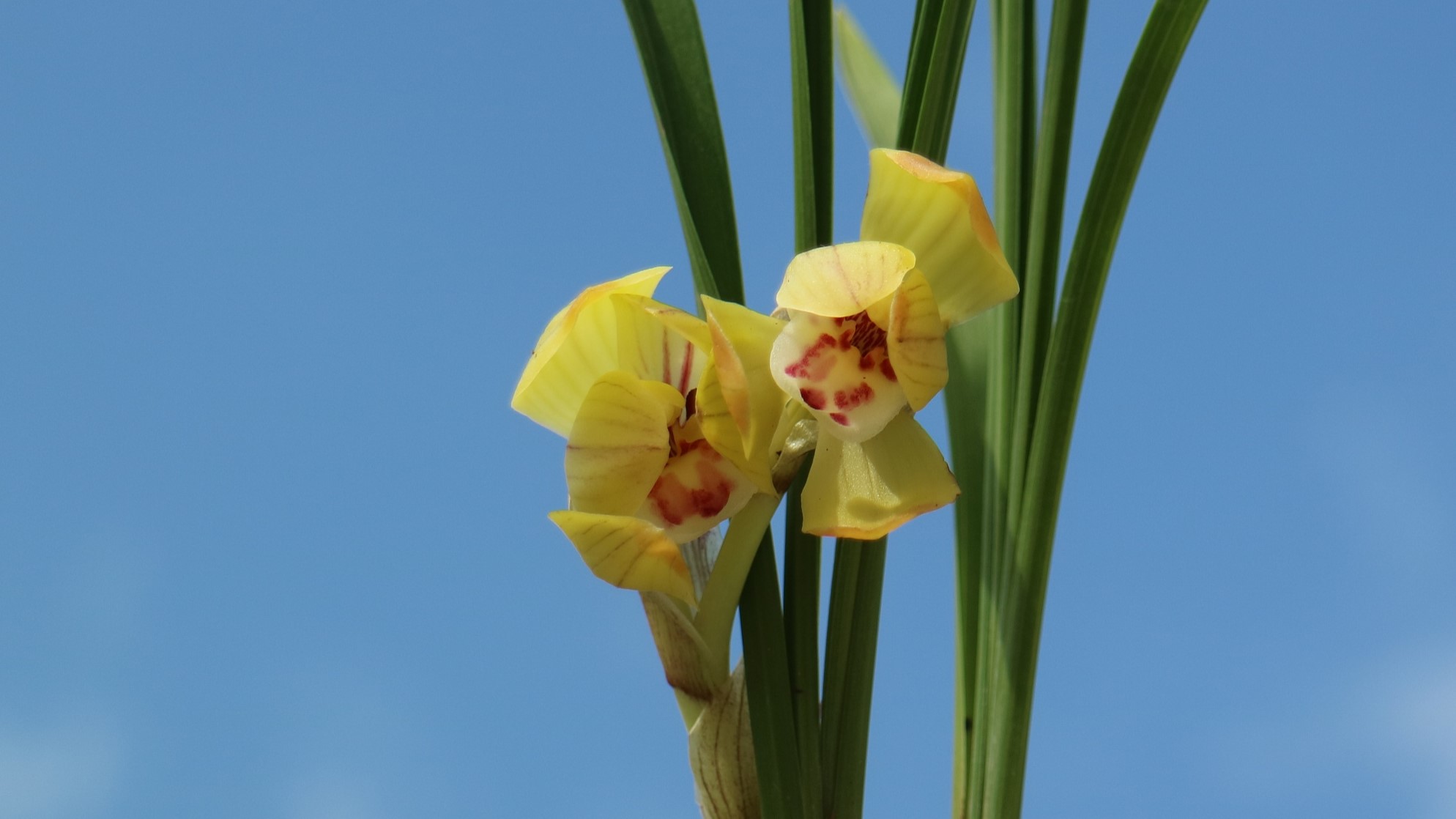
Orchid2024 Dataset
Orchid2024 Dataset
Download link: https://github.com/pengyingshu/orchid2024.
1 Data collection
Chinese Cymbidium orchids, cherished for their deep-rooted cultural significance and significant economic value in China, have spawned a rich tapestry of cultivars. However, these orchid cultivars are facing challenges from insufficient cultivation practices and antiquated techniques, including cultivar misclassification, complex identification, and the proliferation of counterfeit products. Current commercial techniques and academic research primarily emphasize species identification of orchids, rather than delving into that of orchid cultivars within species.
Between October 2022 and January 2024, we systematically collected images of Chinese Cymbidium orchids in accordance with the blooming periods of different species, involving visits to 20 cities across 12 provincial administrative regions in China. The constructed dataset, the Orchid2024 dataset contains 156,630 images spanning 1,269 cultivars and six additional subclasses.
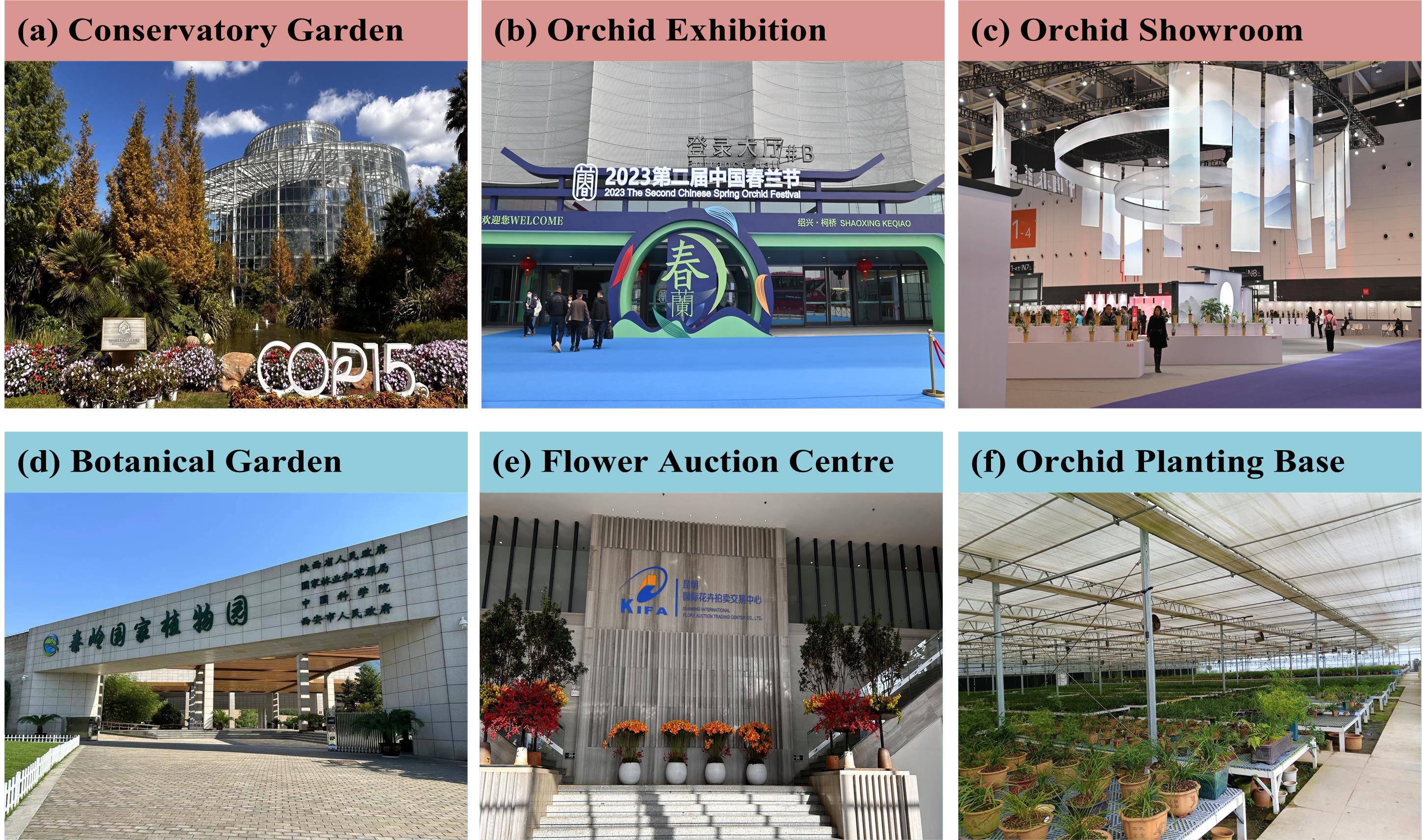
2 Dataset structure
Chinese Cymbidium orchids mentioned in the dataset are a generic term for 8 terrestrial species that originated in China, which belong to the genus Cymbidium within the family Orchidaceae. These 8 species botanically include Cymbidium goeringii, C. faberi, C. ensifolium, C. sinense, C. kanran, and three variants of Cymbidium goeringii, namely, C. var. longibracteatum, C. var. tortisepalum, and C. var. serratum.
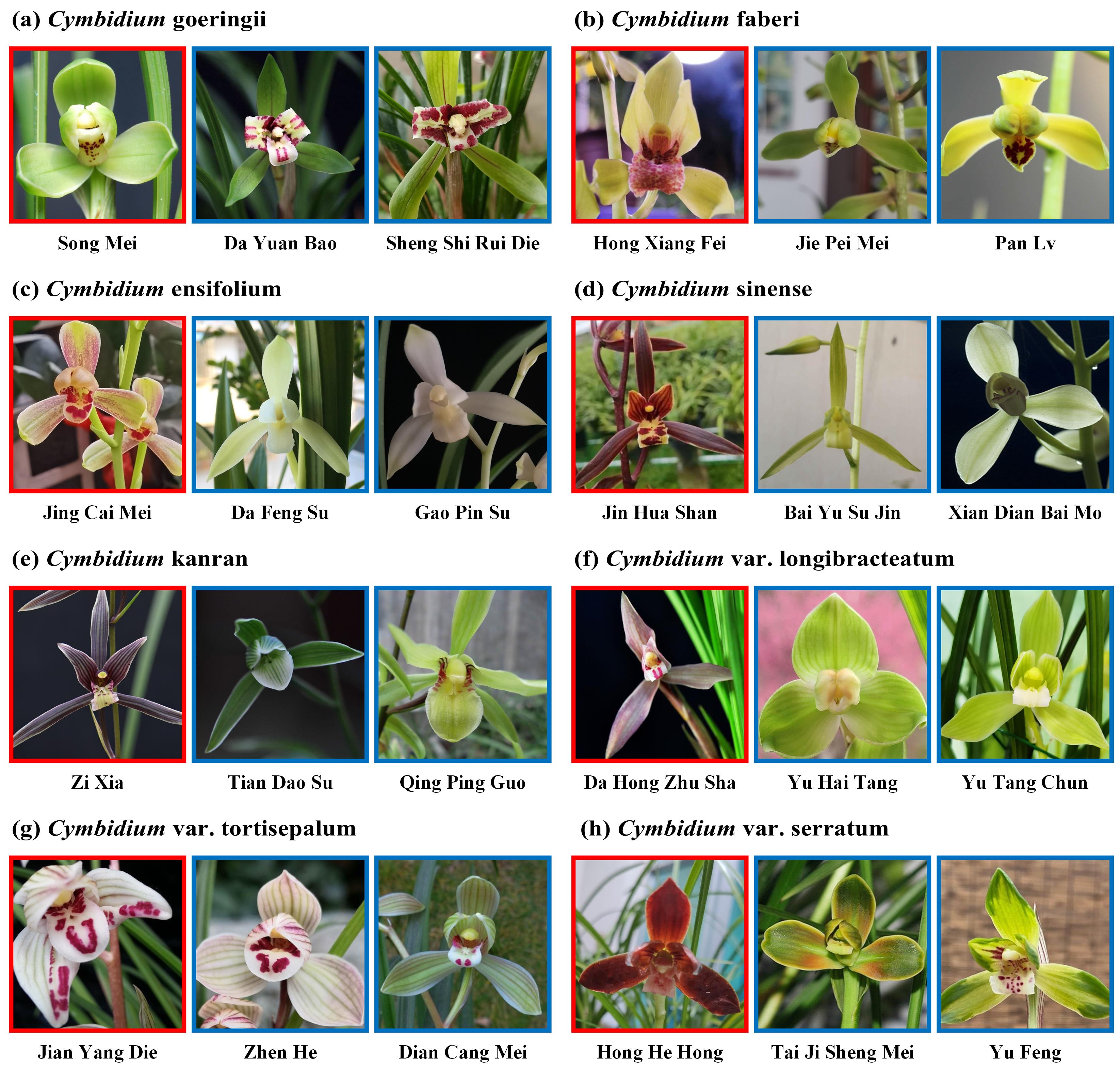
Moreover, six additional categories are introduced into the dataset. These categories include images of orchids outside the genus Cymbidium, which belong to the family Orchidaceae, as well as flower images from outside the Orchidaceae family. Additionally, the categories contain images of four other species within the Cymbidium genus, namely Cymbidium lancifolium, C. hybrid, C. floribundum, and C. szechuanicum.
ID | Cultivar | Image | Cultivar Chinese Name | Specie | Count |
---|---|---|---|---|---|
class0000 | C. goeringii 'Qi Cai Yun Nan' | 七彩云南春兰 | C. goeringii | 21 | |
class0001 | C. goeringii 'Qi Cai Xin Chang' | 七彩新昌春兰 | C. goeringii | 208 | |
class0002 | C. goeringii 'Wan Zi Wai Die' | 万字外蝶春兰 | C. goeringii | 17 | |
class0003 | C. goeringii 'Wan Zi' | 万字春兰 | C. goeringii | 434 | |
class0004 | C. goeringii 'Wan Nian Mei' | 万年梅春兰 | C. goeringii | 42 | |
class0005 | C. goeringii 'Wan Chun Mei' | 万春梅春兰 | C. goeringii | 10 | |
class0006 | C. goeringii 'Wan Tai Mei' | 万泰梅春兰 | C. goeringii | 22 | |
class0007 | C. goeringii 'Wan Hong Su' | 万红素春兰 | C. goeringii | 195 | |
class0008 | C. goeringii 'Wan Qing He' | 万青荷春兰 | C. goeringii | 153 | |
class0009 | C. goeringii 'San Yuan' | 三元春兰 | C. goeringii | 14 |
3 Dataset characteristics
As a fine-grained dataset, the Orchid2024 dataset demonstrates a noticeable imbalance in distribution at both the specie and cultivar levels. This disparity is predominantly attributed to factors such as market demand, breeding complexities, culminating in a long-tail distribution. The quantity of images per category spans from a minimum of 10 to a maximum of 1,387. At the same time, because the dataset involves two granularity levels of species and cultivated varieties under species.
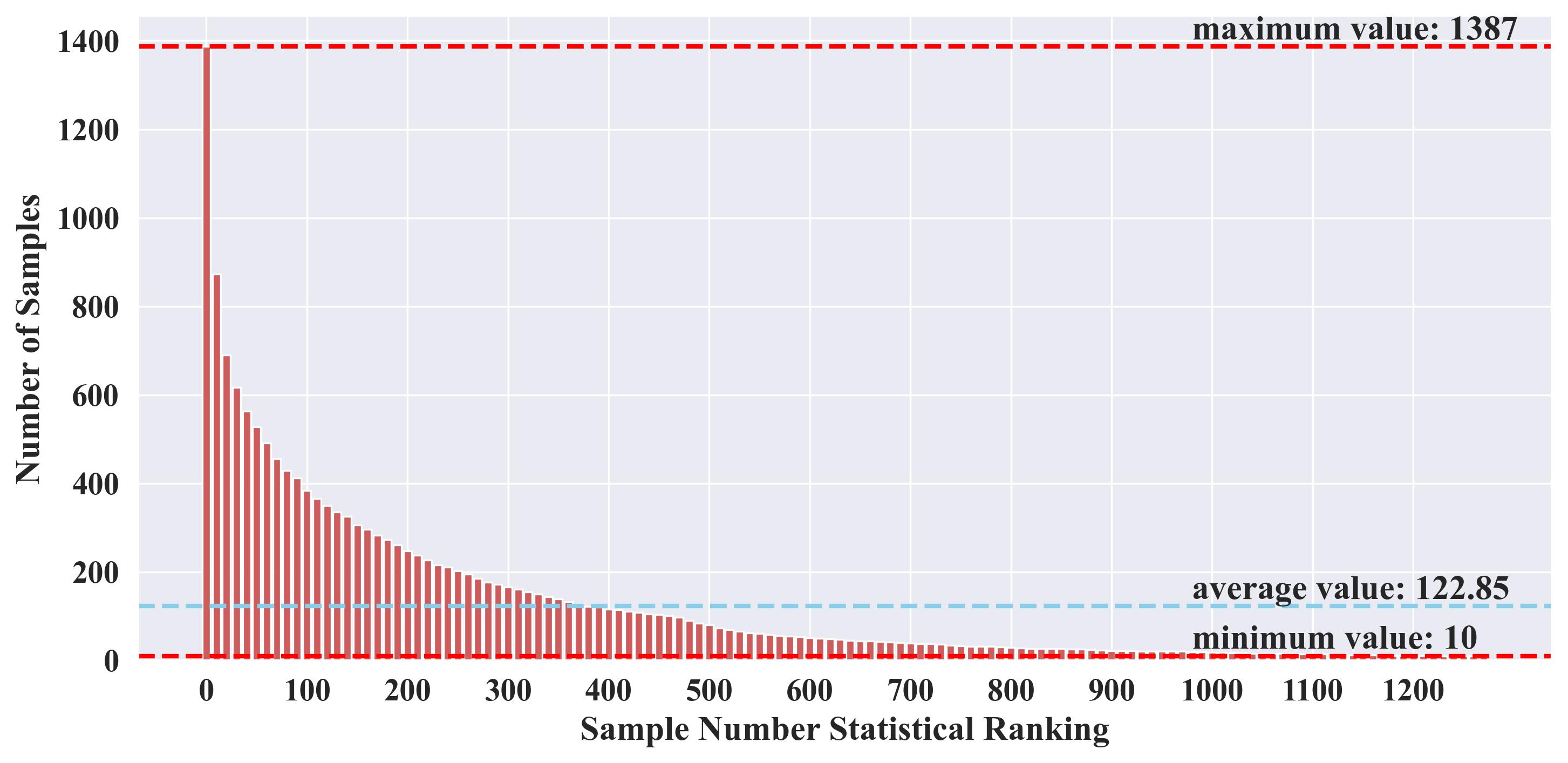
When comparing the Orchid2024 dataset with several popular fine-grained image datasets, it can be observed that currently known famous fine-grained datasets mainly focus on common object classification within the general domain, such as food, animals, and daily necessities. In contrast, the Orchid2024 dataset offers a deep dive into a specific domain, providing fine-grained detail for orchid cultivars classification. Given that orchids exhibit unique characteristics and complex morphology, their distinction and recognition require more meticulous observation and understanding. Furthermore, the Orchid2024 dataset is comparable to the widely used fine-grained datasets currently available in terms of both the size and diversity of the images.
Dataset | Year | Meta-Class | Images | Categories |
---|---|---|---|---|
OxfordFlowers102[1] | 2008 | Flowers | 8,189 | 102 |
CUB200-2011[2] | 2011 | Birds | 11,788 | 200 |
StanfordDogs[3] | 2011 | Dogs | 20,580 | 120 |
Food101[4] | 2014 | Fooddishes | 101,000 | 101 |
DeepFashion[5] | 2016 | Clothes | 800,000 | 1,050 |
Veg200[6] | 2017 | Vegetable | 91,117 | 200 |
Products-10K[7] | 2020 | Retailproducts | 150,000 | 10,000 |
iNat2021[8] | 2021 | Plants&Animals | 3,286,843 | 10,000 |
Orchid2024 | 2024 | Orchidcultivars | 156,630 | 1,275 |
4 Experimental result
Considering the similarity of images across different orchid cultivars, we explored a different route in developing an efficient classification model. We introduce visual parameter-efficient fine-tuning (PEFT) for the orchid cultivar accurately classification. Inspired by recent advancements in language models, PEFT offers a powerful alternative to full-parameter fine-tuning for adapting pre-trained vision models, particularly in resource-constrained scenarios[9].
When comparing the performance of various PEFT methods using the default configuration on the Orchid2024 dataset, LoRA (Low-Rank Adaptation)[10], with a focus on tuning the Q and V parameters, achieves an 85.12% top-1 test accuracy within a ViT/B-16 model. Subsequently, if more fine-tuning parameters are added, that is, when LoRA fine-tunes the query (Q), key (K), value (V), and output (O) layers specifically within a ViT/B-16 model, it achieves an 86.14% top-1 test accuracy, representing the best result in the experiment.
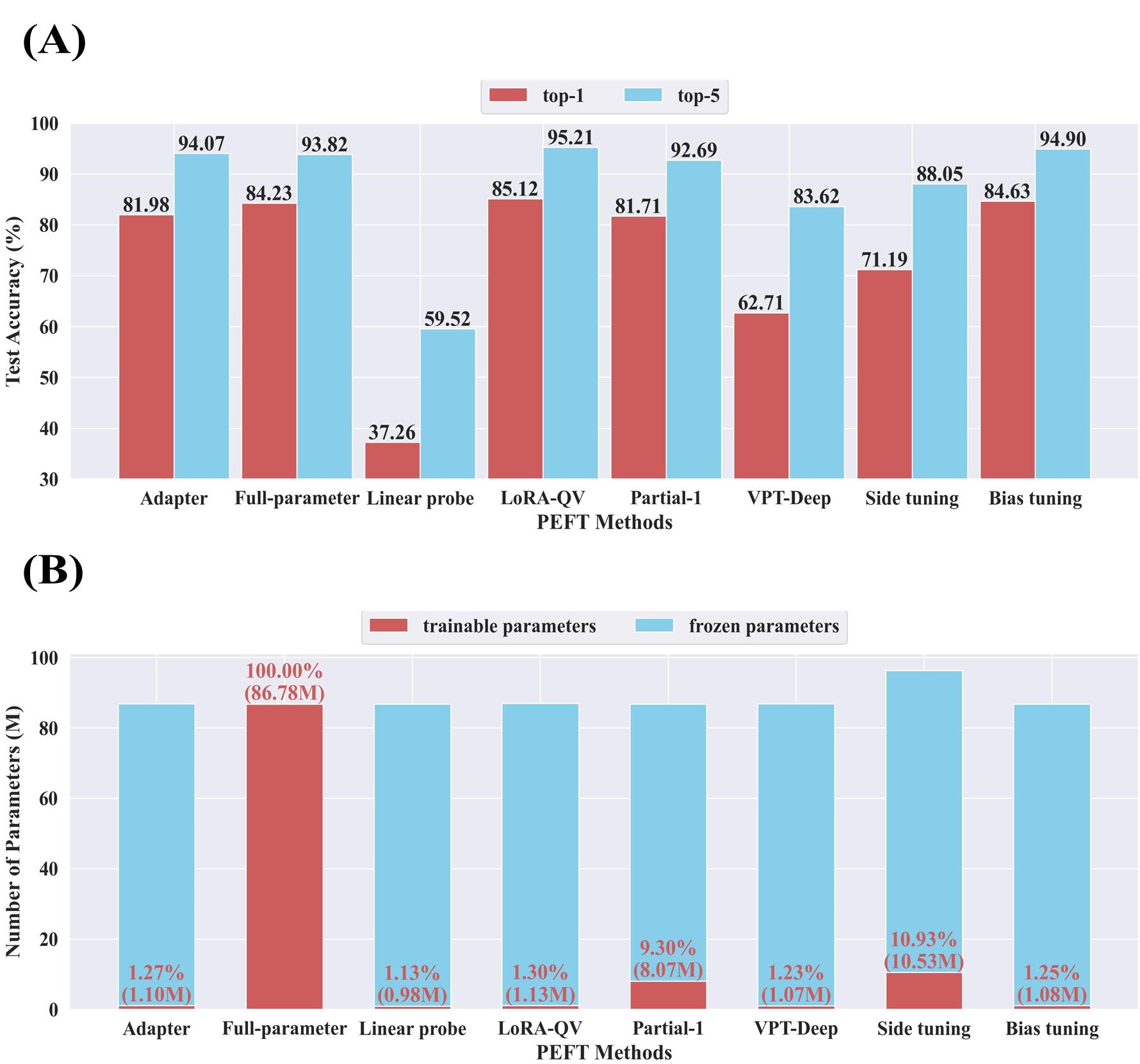
Nilsback, Maria-Elena, and Andrew Zisserman. "Automated flower classification over a large number of classes." 2008 Sixth Indian conference on computer vision, graphics & image processing. IEEE, 2008. ↩︎
Ren, Jie, et al. "Few-Shot Fine-Grained Image Classification: A Comprehensive Review." AI 5.1 (2024): 405-425. ↩︎
Khosla, Aditya, et al. "Novel dataset for fine-grained image categorization: Stanford dogs." Proc. CVPR workshop on fine-grained visual categorization (FGVC). Vol. 2. No. 1. Citeseer, 2011. ↩︎
Bossard, Lukas, Matthieu Guillaumin, and Luc Van Gool. "Food-101–mining discriminative components with random forests." Computer Vision–ECCV 2014: 13th European Conference, Zurich, Switzerland, September 6-12, 2014, Proceedings, Part VI 13. Springer International Publishing, 2014. ↩︎
Liu, Ziwei, et al. "Deepfashion: Powering robust clothes recognition and retrieval with rich annotations." Proceedings of the IEEE conference on computer vision and pattern recognition. 2016. ↩︎
Hou, Saihui, Yushan Feng, and Zilei Wang. "Vegfru: A domain-specific dataset for fine-grained visual categorization." Proceedings of the IEEE international conference on computer vision. 2017. ↩︎
Bai, Yalong, et al. "Products-10k: A large-scale product recognition dataset." arXiv preprint arXiv:2008.10545 (2020). ↩︎
Van Horn, Grant, et al. "Benchmarking representation learning for natural world image collections." Proceedings of the IEEE/CVF conference on computer vision and pattern recognition. 2021. ↩︎
Jia, Menglin, et al. "Visual prompt tuning." European Conference on Computer Vision. Cham: Springer Nature Switzerland, 2022. ↩︎
Yu, Yu, et al. "Low-rank adaptation of large language model rescoring for parameter-efficient speech recognition."2023 IEEE Automatic Speech Recognition and Understanding Workshop (ASRU). IEEE, 2023. ↩︎